Comparative Analysis of Random Forest and Logistic Regression for Heart Attack Risk Prediction
DOI:
https://doi.org/10.57159/jcmm.3.5.24123Keywords:
Heart Attack, Machine learning, Early Detection, Random Forest, Linear RegressionAbstract
Cardiovascular diseases, particularly heart attacks, are leading causes of global mortality, highlighting the need for enhanced early detection and intervention strategies. This study evaluates the effectiveness of two machine learning algorithms—Random Forest (RF) and Logistic Regression (LR)—in predicting heart attack risk using diverse patient data sets. The focus is on uncovering subtle patterns and risk factors that traditional methods may overlook, while also assessing the accuracy and performance of both models. A critical aspect of the study is the interpretability of these algorithms, addressing a significant gap in current research. Additionally, the issue of dataset imbalance, which is prevalent in medical data, is examined, and solutions are proposed to improve model reliability in real-world applications. These findings contribute to the discourse on optimizing machine learning in healthcare, advocating for tailored approaches that balance predictive power with interpretability. By analyzing the strengths and weaknesses of RF and LR in heart attack prediction, this study aims to provide valuable insights for clinicians and researchers, ultimately enhancing decision-making processes in cardiovascular care and interventions.
References
M. Amini, F. Zayeri, and M. Salehi, “Trend analysis of cardiovascular disease mortality, incidence, and mortality-to-incidence ratio: results from global burden of disease study 2017,” BMC Public Health, vol. 21, pp. 1–12, 2021.
E. Radwa, H. Ridha, and B. Faycal, “Deep learning-based approaches for myocardial infarction detection: A comprehensive review of recent advances and emerging challenges,” Medicine in Novel Technology and Devices, p. 100322, 2024.
Y. Kumari, P. Bai, F. Waqar, A. T. Asif, B. Irshad, S. Raj, V. Varagantiwar, M. Kumar, F. Neha, S. Chand, et al., “Advancements in the management of endocrine system disorders and arrhythmias: A comprehensive narrative review,” Cureus, vol. 15, no. 10, 2023.
G. M. Dogheim and A. Hussain, “Patient care through AI-driven remote monitoring: Analyzing the role of predictive models and intelligent alerts in preventive medicine,” Journal of Contemporary Healthcare Analytics, vol. 7, no. 1, pp. 94–110, 2023.
A. Ogunpola, F. Saeed, S. Basurra, A. M. Albarrak, and S. N. Qasem, “Machine learning-based predictive models for detection of cardiovascular diseases,” Diagnostics, vol. 14, no. 2, p. 144, 2024.
S. Zhong, K. Zhang, M. Bagheri, J. G. Burken, A. Gu, B. Li, X. Ma, B. L. Marrone, Z. J. Ren, J. Schrier, et al., “Machine learning: new ideas and tools in environmental science and engineering,” Environmental Science & Technology, vol. 55, no. 19, pp. 12741–12754, 2021.
Z. Noroozi, A. Orooji, and L. Erfannia, “Analyzing the impact of feature selection methods on machine learning algorithms for heart disease prediction,” Scientific Reports, vol. 13, no. 1, p. 22588, 2023.
M. Salmi, D. Atif, D. Oliva, A. Abraham, and S. Ventura, “Handling imbalanced medical datasets: Review of a decade of research,” Artificial Intelligence Review, vol. 57, no. 10, p. 273, 2024.
R. Hasan, “Comparative analysis of machine learning algorithms for heart disease prediction,” in ITM Web of Conferences, vol. 40, p. 03007, EDP Sciences, 2021.
A. Abdellatif, H. Abdellatef, J. Kanesan, C.-O. Chow, J. H. Chuah, and H. M. Gheni, “Improving the heart disease detection and patients’ survival using supervised infinite feature selection and improved weighted random forest,” IEEE Access, vol. 10, pp. 67363–67372, 2022.
M. S. Pathan, A. Nag, M. M. Pathan, and S. Dev, “Analyzing the impact of feature selection on the accuracy of heart disease prediction,” Healthcare Analytics, vol. 2, p. 100060, 2022.
L. Li and Z.-P. Liu, “A connected network-regularized logistic regression model for feature selection,” Applied Intelligence, vol. 52, no. 10, pp. 11672–11702, 2022.
J. Hu and S. Szymczak, “A review on longitudinal data analysis with random forest,” Briefings in Bioinformatics, vol. 24, no. 2, p. bbad002, 2023.
M. Maalouf, “Logistic regression in data analysis: an overview,” International Journal of Data Analysis Techniques and Strategies, vol. 3, no. 3, pp. 281–299, 2011.
T. J. Cleophas, A. H. Zwinderman, T. J. Cleophas, and A. H. Zwinderman, “Bayesian Pearson correlation analysis,” Modern Bayesian Statistics in Clinical Research, pp. 111–118, 2018.
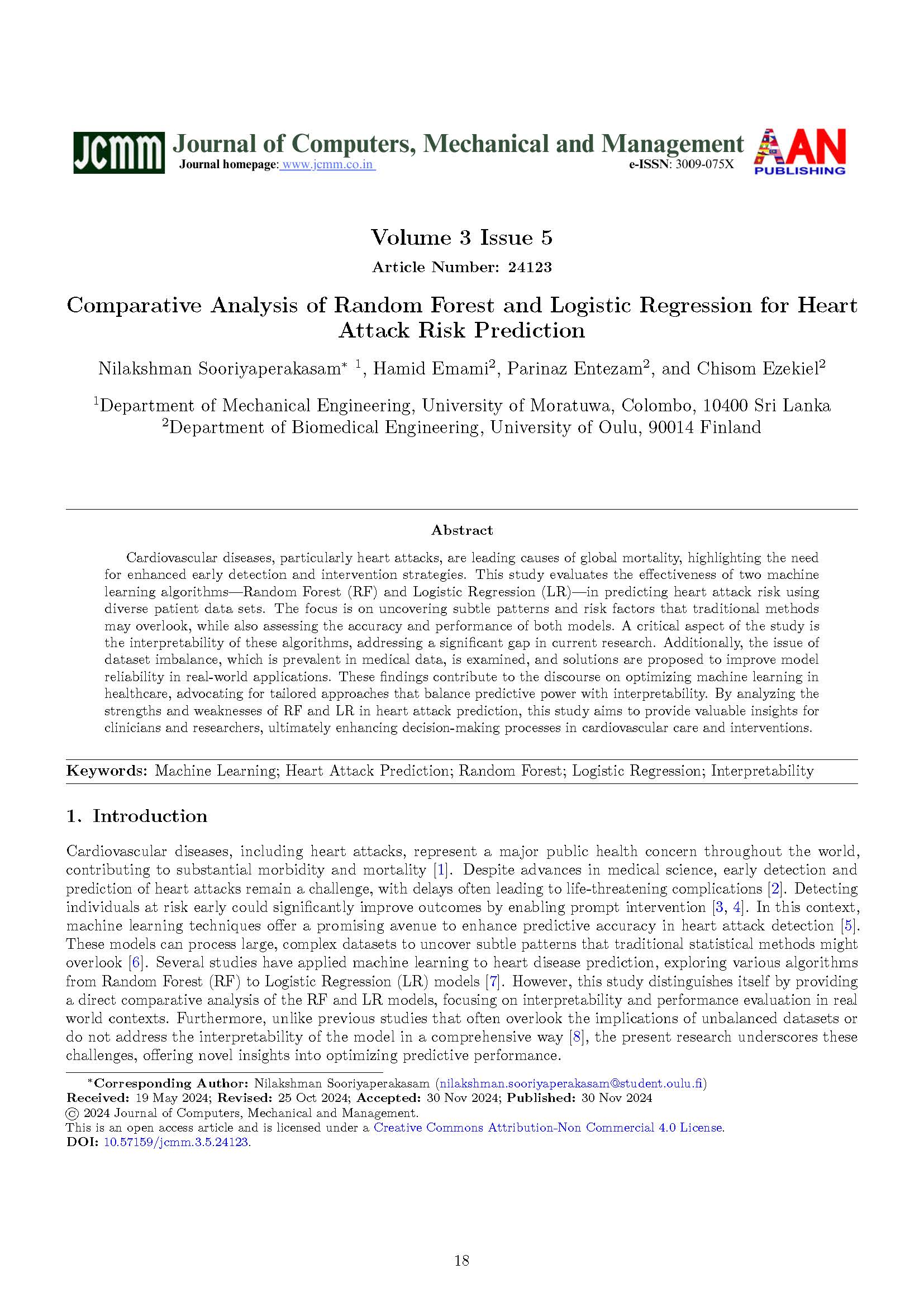
Downloads
Published
How to Cite
Issue
Section
Categories
License
Copyright (c) 2024 Journal of Computers, Mechanical and Management

This work is licensed under a Creative Commons Attribution-NonCommercial 4.0 International License.
The Journal of Computers, Mechanical and Management applies the CC Attribution- Non-Commercial 4.0 International License to its published articles. While retaining copyright ownership of the content, the journal permits activities such as downloading, reusing, reprinting, modifying, distributing, and copying of the articles, as long as the original authors and source are appropriately cited. Proper attribution is ensured by citing the original publication.
Accepted 2024-11-30
Published 2024-11-30