Advancing Artificial Intelligence Adoption and Decision-making with Extended Technology Acceptance Model
DOI:
https://doi.org/10.57159/jcmm.3.4.24137Keywords:
AI Adoption, Technology Acceptance Model (TAM), Organizational Support, Emerging MarketsAbstract
Despite Kuala Lumpur’s push for AI integration, only 23% of businesses have adopted AI, lagging behind the global average of 37%, with 65% still relying on basic data tools and only 10% using advanced analytics. This study investigates the factors influencing AI adoption in Kuala Lumpur's IT sector, focusing on Perceived Ease of Use (PEOU), Perceived Usefulness (PU), and Perceived Organizational Support (POS). Using the Technology Acceptance Model (TAM) and Unified Theory of Acceptance and Use of Technology (UTAUT) as theoretical foundations, this study extends these frameworks by incorporating POS to emphasize the critical role of organizational support in AI adoption. Data from a survey of 340 IT managers were analyzed us- ing PLS-SEM. The results demonstrate that both PEOU and POS significantly impact PU, which in turn influences AI adoption intentions. POS emerged as a vital factor, indicating that organizational support, such as training and resource provision, is key in making AI useful and encouraging its adoption. This research has practical implications for businesses and policymakers. Organizations should focus on improving organizational support mechanisms, particularly through targeted training programs and technical assistance to enhance AI adoption. Policymakers are encouraged to refine initiatives like Industry4WRD by strengthening infrastructure and providing sector-specific support. The study's novelty lies in its focus on emerging markets like Kuala Lumpur, addressing a gap in AI adoption research by exploring the organizational challenges specific to such regions.
References
S. Russell and P. Norvig, Artificial Intelligence: A Modern Approach, 4th ed., Pearson, 2020.
B. Nguyen and D. S. Mutum, “A review of customer relationship management: Successes, advances, pitfalls, and futures,” Business Process Management Journal, vol. 18, no. 3, pp. 400–419, 2012.
S. F. Wamba and S. Akter, “Impact of artificial intelligence on business marketing: A systematic literature review,” Journal of Business Research, vol. 101, pp. 637–645, 2019.
E. Brynjolfsson and A. McAfee, The Second Machine Age: Work, Progress, and Prosperity in a Time of Brilliant Technologies, W.W. Norton & Company, 2014.
Malaysian Digital Economy Corporation, “AI Adoption Survey,” 2020.
PricewaterhouseCoopers, “Data and Analytics Survey: Malaysia,” 2019.
M. A. Boden, Artificial Intelligence: A Very Short Introduction, Oxford University Press, 2018.
Malaysian Investment Development Authority, “Industry4wrd: National policy on industry 4.0,” 2018.
Ministry of International Trade and Industry, “Malaysia’s Artificial Intelligence Roadmap (AI-RMAP),” 2021.
F. D. Davis, “Perceived usefulness, perceived ease of use, and user acceptance of information technology,” MIS Quarterly, vol. 13, no. 3, pp. 319–340, 1989.
V. Venkatesh, M. G. Morris, G. B. Davis, and F. D. Davis, “User acceptance of information technology: Toward a unified view,” MIS Quarterly, vol. 27, no. 3, pp. 425–478, 2003.
M.-P. Gagnon, J. Gagnon, and J. D. Jacob, “Perceived organizational support for technology use as a moderator of the relationship between organizational characteristics and clinician IT acceptance,” BMC Health Services Research, vol. 20, no. 1, pp. 1–10, 2020.
V. Venkatesh and H. Bala, “Technology acceptance model 3 and a research agenda on interventions,” Decision Sciences, vol. 39, no. 2, pp. 273–315, 2008.
V. Venkatesh and F. D. Davis, “A theoretical extension of the technology acceptance model: Four longitudinal field studies,” Management Science, vol. 46, no. 2, pp. 186–204, 2000.
I. Im, S. H. Hong, and M. S. Kang, “An international comparison of technology adoption: Testing the UTAUT model,” Information & Management, vol. 48, no. 1, pp. 1–8, 2011.
Y. K. Dwivedi, D. L. Hughes, E. Ismagilova, G. Aarts, C. Coombs, T. Crick, et al., “Artificial intelligence (AI): Multidisciplinary perspectives on emerging challenges, opportunities, and agenda for research, practice, and policy,” International Journal of Information Management, vol. 57, p. 101994, 2021.
TechBehemoths, “The IT industry in Malaysia: General portrait,” 2023.
EY, “Why Kuala Lumpur is garnering investments from next-gen digital MNCs,” 2024.
ASEAN Access, “Malaysia digitalization and IT sector,” 2023.
DataMites, “What are the top IT companies in Kuala Lumpur?,” 2023.
J. Cohen, Statistical Power Analysis for the Behavioral Sciences, 2nd ed., Lawrence Erlbaum Associates, 1988.
F. Faul, E. Erdfelder, A.-G. Lang, and A. Buchner, “G*Power 3: A flexible statistical power analysis program for the social, behavioral, and biomedical sciences,” Behavior Research Methods, vol. 41, no. 4, pp. 1149–1160, 2009.
R. Eisenberger, R. Huntington, S. Hutchison, and D. Sowa, “Perceived organizational support,” Journal of Applied Psychology, vol. 71, no. 3, pp. 500–507, 1986.
L. R. Shanock et al., “Treating employees well: The value of organizational support theory in human resource management,” The Psychologist-Manager Journal, vol. 22, no. 1, pp. 1–14, 2019.
A. Bryman, Social Research Methods, 5th ed., Oxford University Press, 2016.
J. W. Creswell, Research Design: Qualitative, Quantitative, and Mixed Methods Approaches, 4th ed., SAGE Publications, 2014.
J. F. Hair, G. T. M. Hult, C. M. Ringle, and M. Sarstedt, A Primer on Partial Least Squares Structural Equation Modeling (PLS-SEM), 2nd ed., SAGE Publications, 2017.
J. C. Nunnally and I. H. Bernstein, Psychometric Theory, 3rd ed., McGraw-Hill, 1994.
M. Tavakol and R. Dennick, “Making sense of Cronbach’s alpha,” International Journal of Medical Education, vol. 2, pp. 53–55, 2011.
C. Fornell and D. F. Larcker, “Evaluating structural equation models with unobservable variables and measurement error,” Journal of Marketing Research, vol. 18, no. 1, pp. 39–50, 1981.
J. Henseler, C. M. Ringle, and M. Sarstedt, “A new criterion for assessing discriminant validity in variance-based structural equation modeling,” Journal of the Academy of Marketing Science, vol. 43, no. 1, pp. 115–135, 2015.
S. Geisser, “A predictive approach to the random effect model,” Biometrika, vol. 61, no. 1, pp. 101–107, 1974.
M. Stone, “Cross-validatory choice and assessment of statistical predictions,” Journal of the Royal Statistical Society: Series B (Methodological), vol. 36, no. 2, pp. 111–147, 1974.
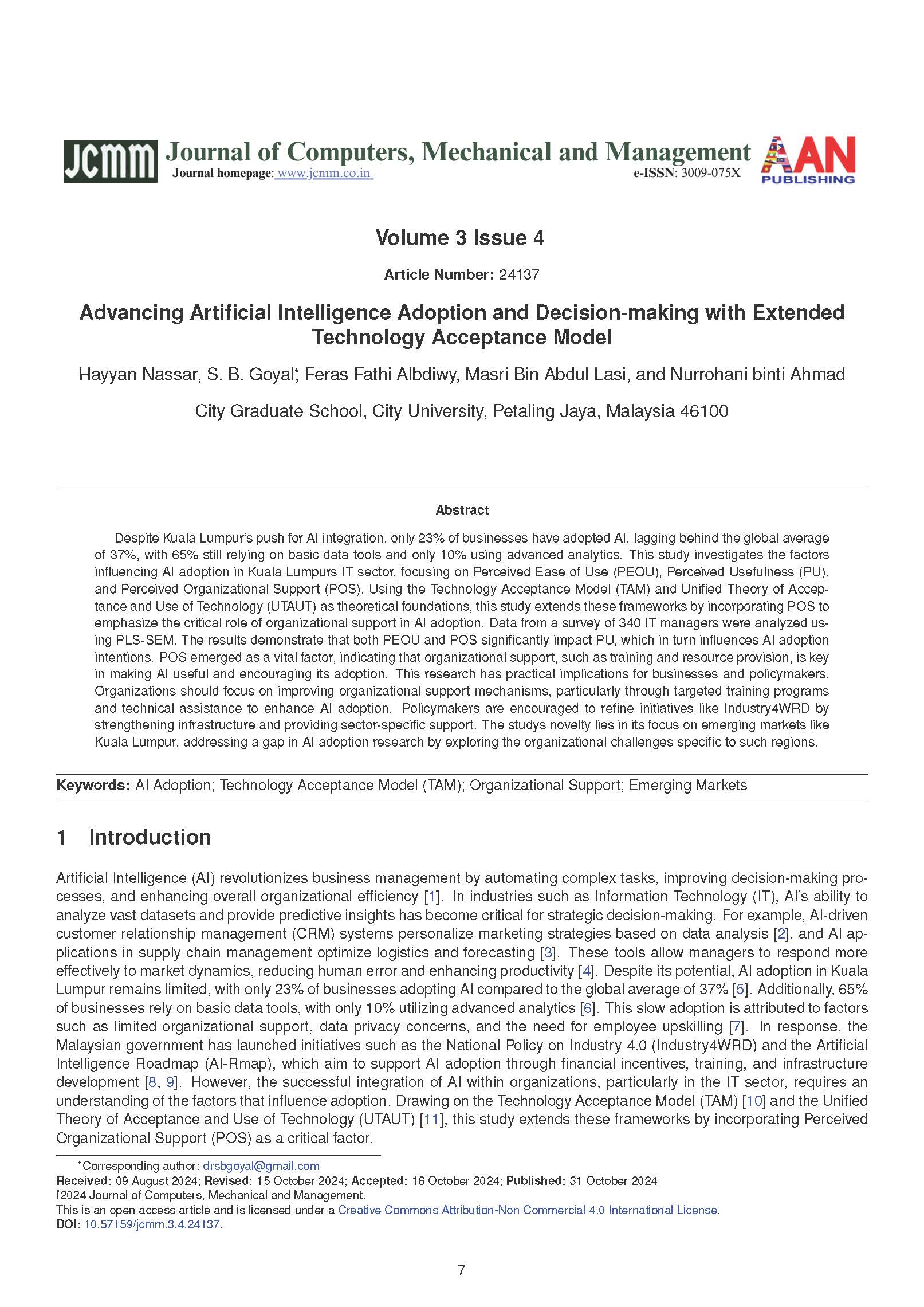
Downloads
Published
How to Cite
License
Copyright (c) 2024 Journal of Computers, Mechanical and Management

This work is licensed under a Creative Commons Attribution-NonCommercial 4.0 International License.
The Journal of Computers, Mechanical and Management applies the CC Attribution- Non-Commercial 4.0 International License to its published articles. While retaining copyright ownership of the content, the journal permits activities such as downloading, reusing, reprinting, modifying, distributing, and copying of the articles, as long as the original authors and source are appropriately cited. Proper attribution is ensured by citing the original publication.
Accepted 2024-10-16
Published 2024-10-31