Astoundingly Smart System Furnishing Ranking of Big Data in Search Engines
DOI:
https://doi.org/10.57159/gadl.jcmm.1.1.22018Keywords:
YAGO dataset, DBpedia, Ontology, Semantic Web, NoSQLAbstract
The abruptly escalating internet is sensational. It inculcates a humungous volume of big data, which is obsolete and tedious to manage, scrutinize, analyze and perform operations upon them in conventional ways. Big data has thus expedited the search and retrieval of information, necessitating the development of contemporary search algorithms to aid this process. However, the primary hindrance for the first and second generations of conventional search engines was the syntax of keywords devoid of semantic meaning and the lack of a knowledge base that linked disparate web material. This article presents a framework based on trendy technologies, specifically Extracting, Transforming and Integrating (ETI) processes, ontology graphs, and indexing Resource Description Framework (RDF) using the wide-column Not only Structured Query Language (NoSQL) method. The most significant contribution in this regard is developing a mathematical model to compute the similarity score between a query and stored RDF documents using semantic relations. Numerous operations were carried out to evaluate the effectiveness of the proposed methodology in installing data sources, such as DBpedia and YAGO dataset. Insofar as experimental results are concerned, the suggested model achieves greater precision than other comparable systems.
References
C. Gavankar, T. Bhosale, D. Gunda, A. Chavan, and S. Hassan, A Comparative Study of Semantic Search Systems, in 2020 International Conference on Computer Communication and Informatics (ICCCI), Jan. 2020, pp. 1–7.
Z. Pan, Optimization of Information Retrieval Algorithm for Digital Library Based on Semantic Search Engine, in 2020 International Conference on Computer Engineering and Application (ICCEA), Mar. 2020, pp. 364–367.
Dramilio, C. Faustine, S. Sanjaya, and B. Soewito, The Effect and Technique in Search Engine Optimization, in 2020 International Conference on Information Management and Technology (ICIMTech), Aug. 2020, pp. 348–353.
O. Chergui, A. Begdouri, and D. Leclet-Groux, A knowledge-based approach for keywords modeling into a semantic graph, “International Journal of Information Science & Technology-iJIST,” 2 (1), pp. 2550–5114, 2018.
M. N. Asim, M. Wasim, M. U. Ghani Khan, N. Mahmood, and W. Mahmood, The Use of Ontology in Retrieval: A Study on Textual, Multilingual, and Multimedia Retrieval, “IEEE Access,” 7, pp. 21662–21686, 2019.
M. M. El-Gayar, N. E. Mekky, A. Atwan, and H. Soliman, Enhanced Search Engine Using Proposed Framework and Ranking Algorithm Based on Semantic Relations, “IEEE Access,” 7, pp. 139337–139349, 2019.
A. Nadeem, M. Hussain, and A. Iftikhar, New Technique to Rank Without Off Page Search Engine Optimization, in 2020 IEEE 23rd International Multitopic Conference (INMIC), Nov. 2020, pp. 1–6.
M. M. El-Gayar, N. E. Mekky, A. Atwan, and H. Soliman, A Novel Knowledge-based Semantic Search Engine, “International journal of simulation: systems, science & technology,” 20 (5), pp. 9.1-9.9, 2019.
Y. S. Negi and S. Kumar, A Comparative Analysis of Keyword- and Semantic-Based Search Engines, in Advances in Intelligent Systems and Computing, 243, 2014, pp. 727–736.
R. Prasad and S. Agarwal, Comparative Study of Big Data Computing and Storage Tools: A Review, “International Journal of Database Theory and Application,” 9 (1), pp. 45–66, 2016.
Singh and C. K. Reddy, A survey on platforms for big data analytics, “Journal of Big Data,” 2 (1), p. 8, 2015.
V. Bevilacqua, V. Santarcangelo, A. Magarelli, A. Bianco, G. Mastronardi, and E. Cascini, A Semantic Search Framework for Document Retrievals (Literature, Art and History) Based on Thesaurus Multiwordnet Like, in Lecture Notes in Computer Science (including subseries Lecture Notes in Artificial Intelligence and Lecture Notes in Bioinformatics), 6838 LNCS, 2011, pp. 456–463.
Y. Qu and G. Cheng, Falcons Concept Search: A Practical Search Engine for Web Ontologies, “IEEE Transactions on Systems, Man, and Cybernetics - Part A: Systems and Humans,” 41 (4), pp. 810–816, 2011.
L. Ding et al., Swoogle Bibliographic Search, “Proceedings of the Thirteenth ACM conference on Information and knowledge management - CIKM ’04,” p. 652, 2004.
A. Hogan, A. Harth, Jj. Umbrich, S. Kinsella, A. Polleres, and S. Decker, Searching and Browsing Linked Data with SWSE: The Semantic Web Search Engine, “SSRN Electronic Journal,” 2011.
Zaveri, D. Kontokostas, U. Leipzig, and S. Hellmann, Linked Data Quality of DBpedia , Freebase, OpenCyc, Wikidata, and YAGO, “Semantic Web,” 0 (0), pp. 1–53, 2017.
Fatima, C. Luca, and G. Wilson, New Framework for Semantic Search Engine, in 2014 UKSim-AMSS 16th International Conference on Computer Modelling and Simulation, Mar. 2014, pp. 446–451.
Oussous, F.-Z. Benjelloun, A. Ait Lahcen, and S. Belfkih, Big Data technologies: A survey, “Journal of King Saud University - Computer and Information Sciences,” 30 (4), pp. 431–448, 2018.
K. Shvachko, H. Kuang, S. Radia, and R. Chansler, The Hadoop Distributed File System, in 2010 IEEE 26th Symposium on Mass Storage Systems and Technologies (MSST), May 2010, pp. 1–10.
Vohra, Using Apache HBase, “Pro Docker,” pp. 141–150, 2016.
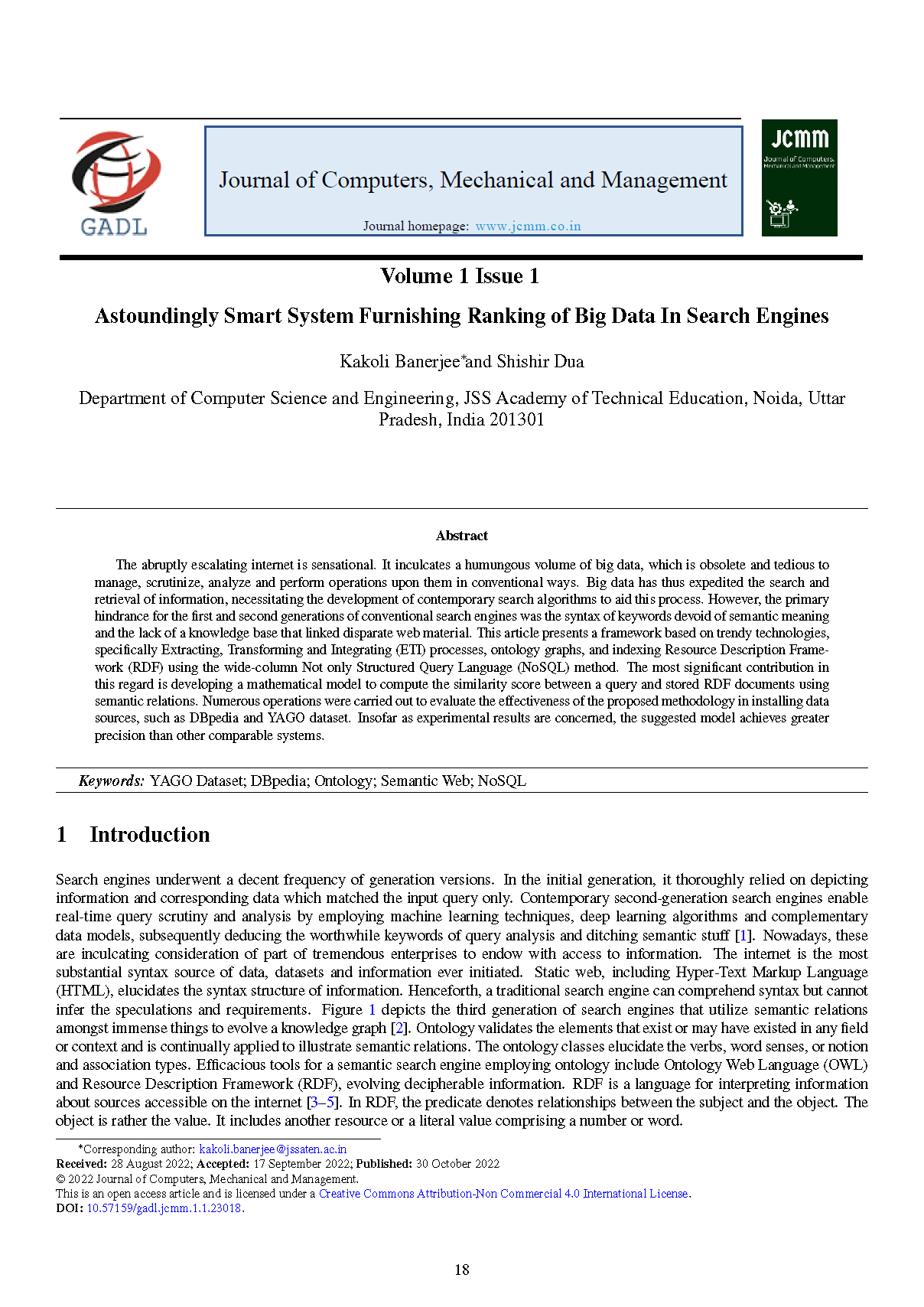
Downloads
Published
How to Cite
Issue
Section
License
Copyright (c) 2022 Journal of Computers, Mechanical and Management

This work is licensed under a Creative Commons Attribution-NonCommercial 4.0 International License.
The Journal of Computers, Mechanical and Management applies the CC Attribution- Non-Commercial 4.0 International License to its published articles. While retaining copyright ownership of the content, the journal permits activities such as downloading, reusing, reprinting, modifying, distributing, and copying of the articles, as long as the original authors and source are appropriately cited. Proper attribution is ensured by citing the original publication.