Precision Diagnosis of Diabetic Retinopathy Using Exudate-Focused SVM Models
DOI:
https://doi.org/10.57159/jcmm.4.1.25148Keywords:
Diabetic Retinopathy, Digital Image Processing, Machine Learning, Support Vector MachinesAbstract
The global concern over diabetes-related eye diseases continues to grow significantly. Diabetic retinopathy, caused by heightened glucose levels in retinal capillaries, leads to vision clouding and eventual blindness. Early detection through regular screening enables intervention with medication, preventing further vision deterioration. Therefore, this study introduces a smart application that utilizes digital retinal image processing to aid in the prompt identification of diabetic retinopathy. The application streamlines the analysis of eye images, with the goal of automatically classifying the severity of diabetic retinopathy. Through initial image processing, specific features such as blood vessels, microaneurysms, and hard exudates are identified and extracted for classification using a support vector machine (SVM). Evaluation performed on a dataset of 400 retinal images graded on a 4-grade scale of non-proliferative diabetic retinopathy achieved a maximum sensitivity rate of 95%. This application holds significant potential for enabling timely intervention in the treatment of diabetic retinopathy by healthcare professionals. Additionally, the AI-driven approach proposed in this study empowers patients to easily access support services, while providing physicians and researchers with advanced tools for analyzing and predicting diabetic retinopathy data. The resulting reports play a crucial role in assessing the severity of the disease in affected individuals.
References
R. Manikandan, A. V. Anand, and G. D. Muthumani, “Phytochemical and in vitro anti-diabetic activity of methanolic extract of Psidium guajava leaves,” International Journal of Current Microbiology and Applied Sciences, vol. 2, no. 2, pp. 15–19, 2013.
T. N. Crawford, D. V. Alfaro III, J. B. Kerrison, E. P. Jablon, et al., “Diabetic retinopathy and angiogenesis,” Current Diabetes Reviews, vol. 5, no. 1, pp. 8–13, 2009.
I. Qureshi, J. Ma, and Q. Abbas, “Recent development on detection methods for the diagnosis of diabetic retinopathy,” Symmetry, vol. 11, no. 6, p. 749, 2019.
N. Asiri, M. Hussain, F. Al Adel, and N. Alzaidi, “Deep learning based computer-aided diagnosis systems for diabetic retinopathy: A survey,” Artificial Intelligence in Medicine, vol. 99, p. 101701, 2019.
I. S. Rajesh, B. M. Reshmi, A. B. Malakreddy, and S. Bilakeri, “Automatic detection of hard exudate in color retinal fundus image and diabetic maculopathy grading,” IEEE Access, 2025.
D. Thulkar, R. Daruwala, and N. Sardar, “An integrated system for detection exudates and severity quantification for diabetic macular edema,” Journal of Medical and Biological Engineering, vol. 40, pp. 798–820, 2020.
N. K. Gahir and M. Shah, “Anatomy of the eye and the healthy fundus,” in Diabetic Retinopathy: Screening to Treatment 2E (ODL), Oxford University Press, p. 13, 2020.
R. J. Chalakkal, W. H. Abdulla, and S. C. Hong, “Fundus retinal image analyses for screening and diagnosing diabetic retinopathy, macular edema, and glaucoma disorders,” in Diabetes and Fundus OCT, Elsevier, pp. 59–111, 2020.
D. S. W. Ting, G. C. M. Cheung, and T. Y. Wong, “Diabetic retinopathy: Global prevalence, major risk factors, screening practices and public health challenges: A review,” Clinical & Experimental Ophthalmology, vol. 44, no. 4, pp. 260–277, 2016.
S. B. Junior and D. Welfer, “Automatic detection of microaneurysms and hemorrhages in color eye fundus images,” International Journal of Computer Science & Information Technology, vol. 5, no. 5, p. 21, 2013.
S. S. Rahim, C. Jayne, V. Palade, and J. Shuttleworth, “Automatic detection of microaneurysms in colour fundus images for diabetic retinopathy screening,” Neural Computing and Applications, vol. 27, pp. 1149–1164, 2016.
S. S. Rahim, V. Palade, J. Shuttleworth, and C. Jayne, “Automatic screening and classification of diabetic retinopathy fundus images,” in Engineering Applications of Neural Networks: 15th International Conference, EANN 2014, Sofia, Bulgaria, September 5–7, 2014. Proceedings 15, Springer, pp. 113–122, 2014.
B. Antal and A. Hajdu, “Improving microaneurysm detection in color fundus images by using context-aware approaches,” Computerized Medical Imaging and Graphics, vol. 37, no. 5–6, pp. 403–408, 2013.
A. Sopharak, B. Uyyanonvara, and S. Barman, “Fine microaneurysm detection from non-dilated diabetic retinopathy retinal images using a hybrid approach,” in Proceedings of the World Congress on Engineering, vol. 2, 2012.
K. M. Adal, D. Sidibé, S. Ali, E. Chaum, T. P. Karnowski, and F. Mériaudeau, “Automated detection of microaneurysms using scale-adapted blob analysis and semi-supervised learning,” Computer Methods and Programs in Biomedicine, vol. 114, no. 1, pp. 1–10, 2014.
R. A. Welikala, J. Dehmeshki, A. Hoppe, V. Tah, S. Mann, T. H. Williamson, and S. A. Barman, “Automated detection of proliferative diabetic retinopathy using a modified line operator and dual classification,” Computer Methods and Programs in Biomedicine, vol. 114, no. 3, pp. 247–261, 2014.
S. K. Yadav, S. Kumar, B. Kumar, and R. Gupta, “Comparative analysis of fundus image enhancement in detection of diabetic retinopathy,” in 2016 IEEE Region 10 Humanitarian Technology Conference (R10-HTC), IEEE, pp. 1–5, 2016.
W. Zhou, C. Wu, D. Chen, Y. Yi, and W. Du, “Automatic microaneurysm detection using the sparse principal component analysis-based unsupervised classification method,” IEEE Access, vol. 5, pp. 2563–2572, 2017.
A. Deka and K. K. Sarma, “SVD and PCA features for ANN based detection of diabetes using retinopathy,” in Proceedings of the CUBE International Information Technology Conference, pp. 38–41, 2012.
P. Alexopoulos, C. Madu, G. Wollstein, and J. S. Schuman, “The development and clinical application of innovative optical ophthalmic imaging techniques,” Frontiers in Medicine, vol. 9, p. 891369, 2022.
C. Schiborn and M. B. Schulze, “Precision prognostics for the development of complications in diabetes,” Diabetologia, vol. 65, no. 11, pp. 1867–1882, 2022.
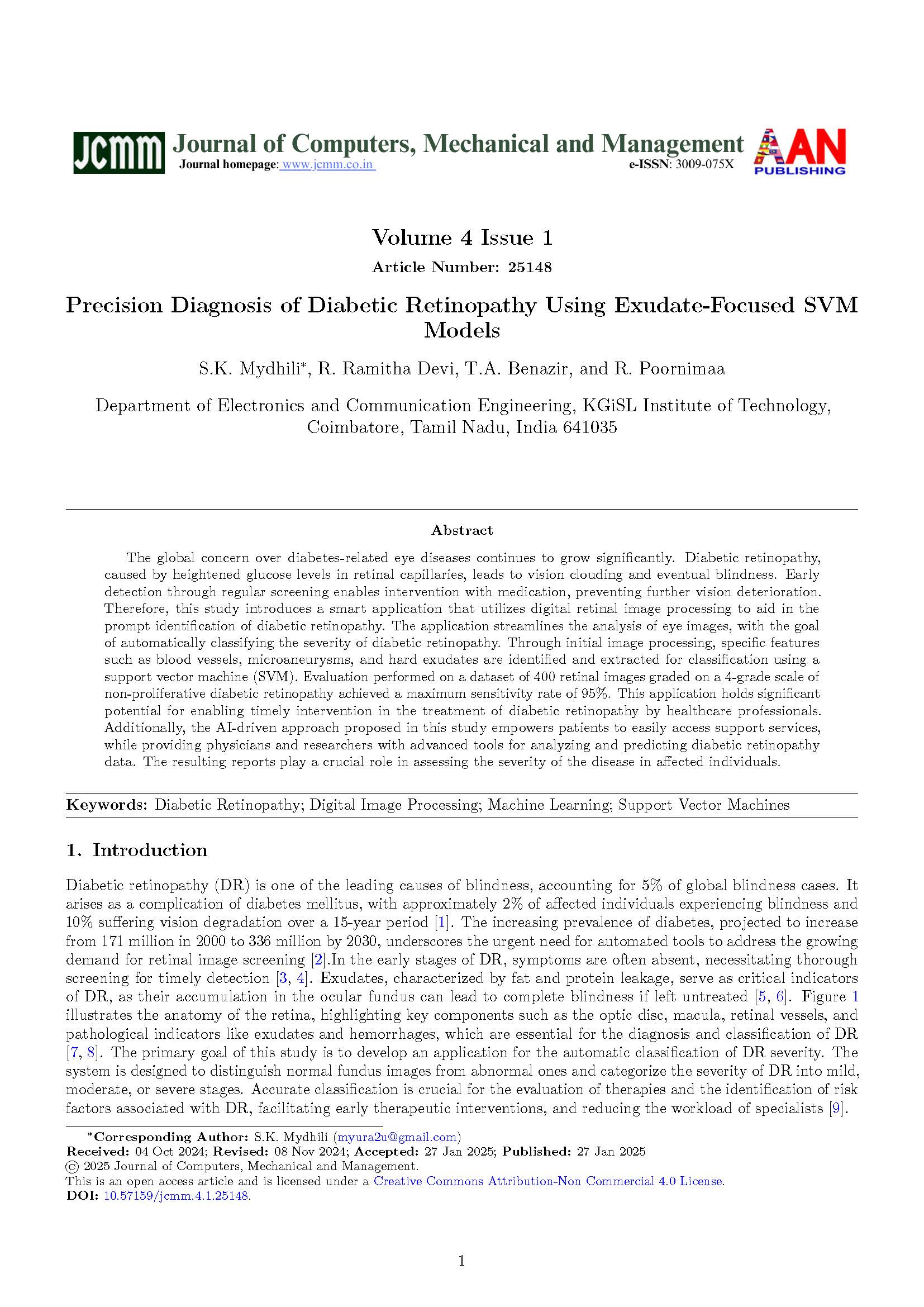
Downloads
Published
How to Cite
Issue
Section
Categories
License
Copyright (c) 2025 Journal of Computers, Mechanical and Management

This work is licensed under a Creative Commons Attribution-NonCommercial 4.0 International License.
The Journal of Computers, Mechanical and Management applies the CC Attribution- Non-Commercial 4.0 International License to its published articles. While retaining copyright ownership of the content, the journal permits activities such as downloading, reusing, reprinting, modifying, distributing, and copying of the articles, as long as the original authors and source are appropriately cited. Proper attribution is ensured by citing the original publication.
Accepted 2025-01-26
Published 2025-01-27