The Study of the Value of π Probability Sampling by Testing Hypothesis and Experimentally
DOI:
https://doi.org/10.57159/gadl.jcmm.3.1.240101Keywords:
Random Number, Triplets, Non-parametric Tests, Friedman’s Test, Chi-square TestAbstract
This study evaluated the value of π using the Monte Carlo Simulation Method and compared the results with experimental values. The experimental value of π was determined by considering a unit circle |z| = 1 centered at the origin, inscribed within a square with vertices (0, 0), (1, 0), (1, 1), and (0, 1). Points were randomly generated within the square, where points satisfying |z| ≤ 1 lay within the circle, and those with |z| ≥ 1 lay outside the circle but within the square. By selecting large numbers of random pairs and determining their positions relative to the circle, the ratio π = 4n/N was calculated, where N was the total number of points and n was the number of points within the circle. Larger sample sizes yielded values of π closer to the true value. The distribution of Monte Carlo Simulation results, using 20 triplets of random numbers, was examined with non-parametric tests such as Friedman’s Test. Ranks were assigned to the 20 random numbers row-wise for each triplet. The null hypothesis, asserting that all triplets had identical effects, was tested and showed significant differences at the 5% level. Additionally, the distribution was tested for goodness of fit using a Chi-Square Test at a 5% significance level. Results indicated that the triplets of random numbers conformed to the expected distribution.References
Dodge, Y., "Statistical data analysis and inference," Elsevier, 2014.
Ismay, C., and Albert Y. Kim, "Statistical Inference via Data Science," 2019.
Milton, J.S., and Arnold, J.C., "Introduction to Probability and Statistics," 2007.
Johnson, R.A., and Gupta, C.B., "Probability and Statistics for Engineers," 2007.
Chandrasekaran, A., and Kavitha, G., "Probability Statistics Random Processes and Queuing Theory," 2014.
Bruce, P., and Andrew Bruce, "Practical Statistics for Data Scientists," 2017.
Rice, J.A., "Mathematical Statistics and Data Analysis," Thomson Higher Education, 2013.
Liu, Z., Blasch, F., and Jhon, V., "Statistical comparison of image fusion algorithms recommendations," Inf. Fusion, 2017.
Couch, S., Kazan, Z., Shi, K., Bray, A., and Groce, A., "Differentially private nonparametric hypothesis testing," Proc. of the ACM Conf. Comput. Commun. Secur., 2019.
García, S., Fernández, A., Luengo, J., and Herrera, F., "Advanced non-parametric tests for multiple comparisons in the design of experiments in computational intelligence and data mining: experimental analysis of power," Inf. Sci., 2010.
Pawar, S.D., and Shirke, D.T., "Nonparametric tests for multivariate multi-sample locations based on data depth," J. Stat. Comput. Simul., 2019.
Derrac, J., García, S., Hui, S., Suganthan, P.N., and Herrera, F., "Analyzing convergence performance of evolutionary algorithms: a statistical approach," Inf. Sci., 2014.
Demšar, J., "Statistical comparisons of classifiers over multiple data sets," J. Mach. Learn. Res., vol. 7, no. 1, 2006.
Steel, R.G.D., "A multiple comparison sign test: treatments versus control," J. Am. Stat. Assoc., 1959.
Doksum, K., "Robust procedures for some linear models with one observation per cell," Ann. Math. Stat., 1967.
Chandra, T.B., Verma, K., Singh, B.K., Jain, D., and Netam, S.S., "Coronavirus disease (COVID-19) detection in chest X-Ray images using majority voting-based classifier ensemble," Expert Syst. Appl., 2021.
De Gregorio, M., and Giordano, M., "An experimental evaluation of weightless neural networks for multi-class classification," Appl. Soft Comput. J., 2018.
Pulgar, F.J., Charte, F., Rivera, A.J., and Del Jesus, M.J., "Choosing the proper autoencoder for feature fusion based on data complexity and classifiers: analysis, tips and guidelines," Inf. Fusion, 2020.
Shi, S., Ding, S., Zhang, Z., and Jia, W., "Energy-based structural least squares MBSVM for classification," Appl. Intell., 2020.
Liu, J., "Fuzzy support vector machine for imbalanced data with borderline noise," Fuzzy Sets Syst., 2020.
Petrović, M., Miljković, Z., and Jokić, A., "A novel methodology for optimal single mobile robot scheduling using whale optimization algorithm," Appl. Soft Comput. J., 2019.
O’Gorman, T.W., "A comparison of the F-test, Friedman’s test, and several aligned rank tests for the analysis of randomized complete blocks," J. Agric. Biol. Environ. Stat., 2001.
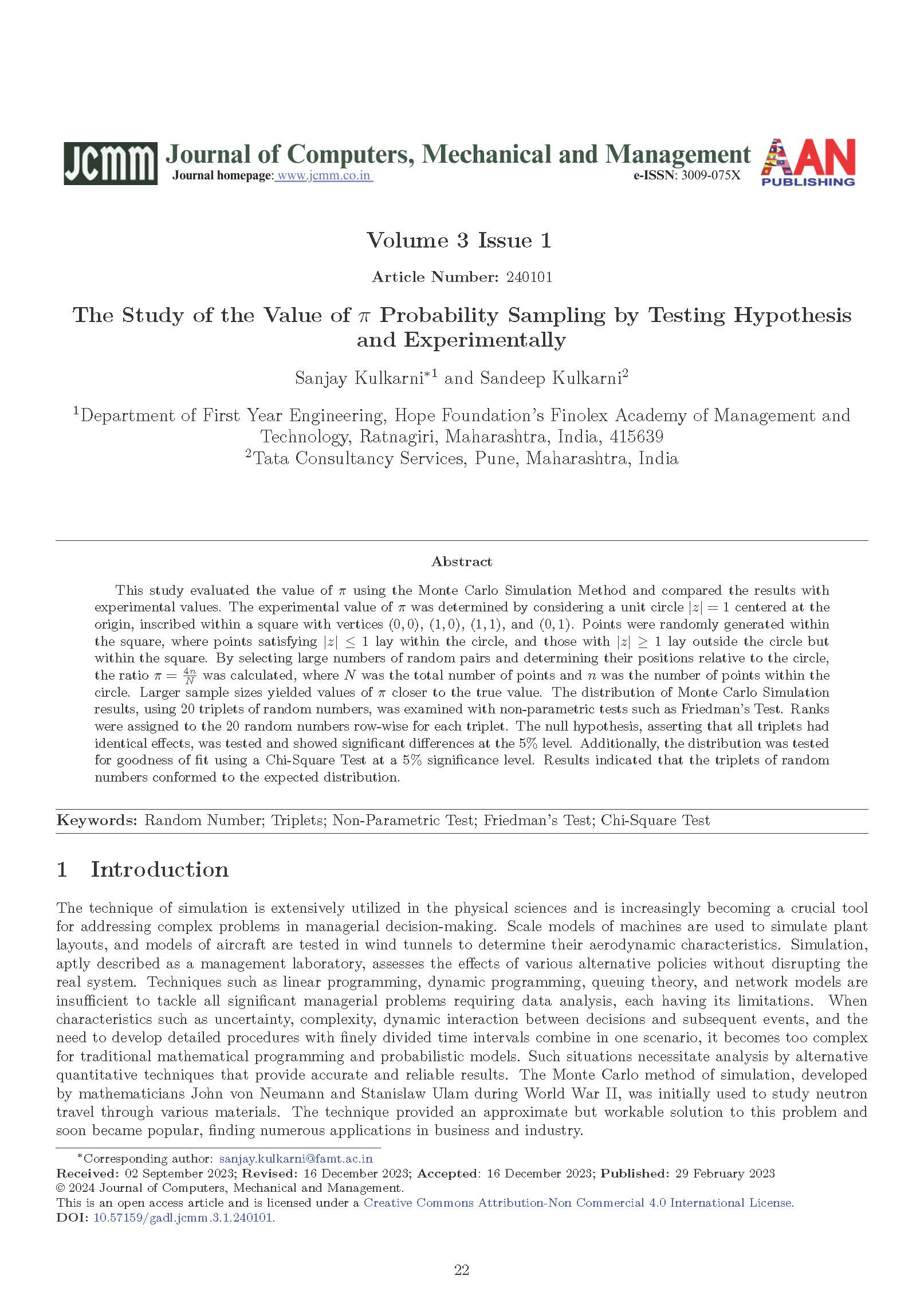
Downloads
Published
How to Cite
Issue
Section
License
Copyright (c) 2023 Journal of Computers, Mechanical and Management

This work is licensed under a Creative Commons Attribution-NonCommercial 4.0 International License.
The Journal of Computers, Mechanical and Management applies the CC Attribution- Non-Commercial 4.0 International License to its published articles. While retaining copyright ownership of the content, the journal permits activities such as downloading, reusing, reprinting, modifying, distributing, and copying of the articles, as long as the original authors and source are appropriately cited. Proper attribution is ensured by citing the original publication.
Accepted 2023-12-16
Published 2024-02-29